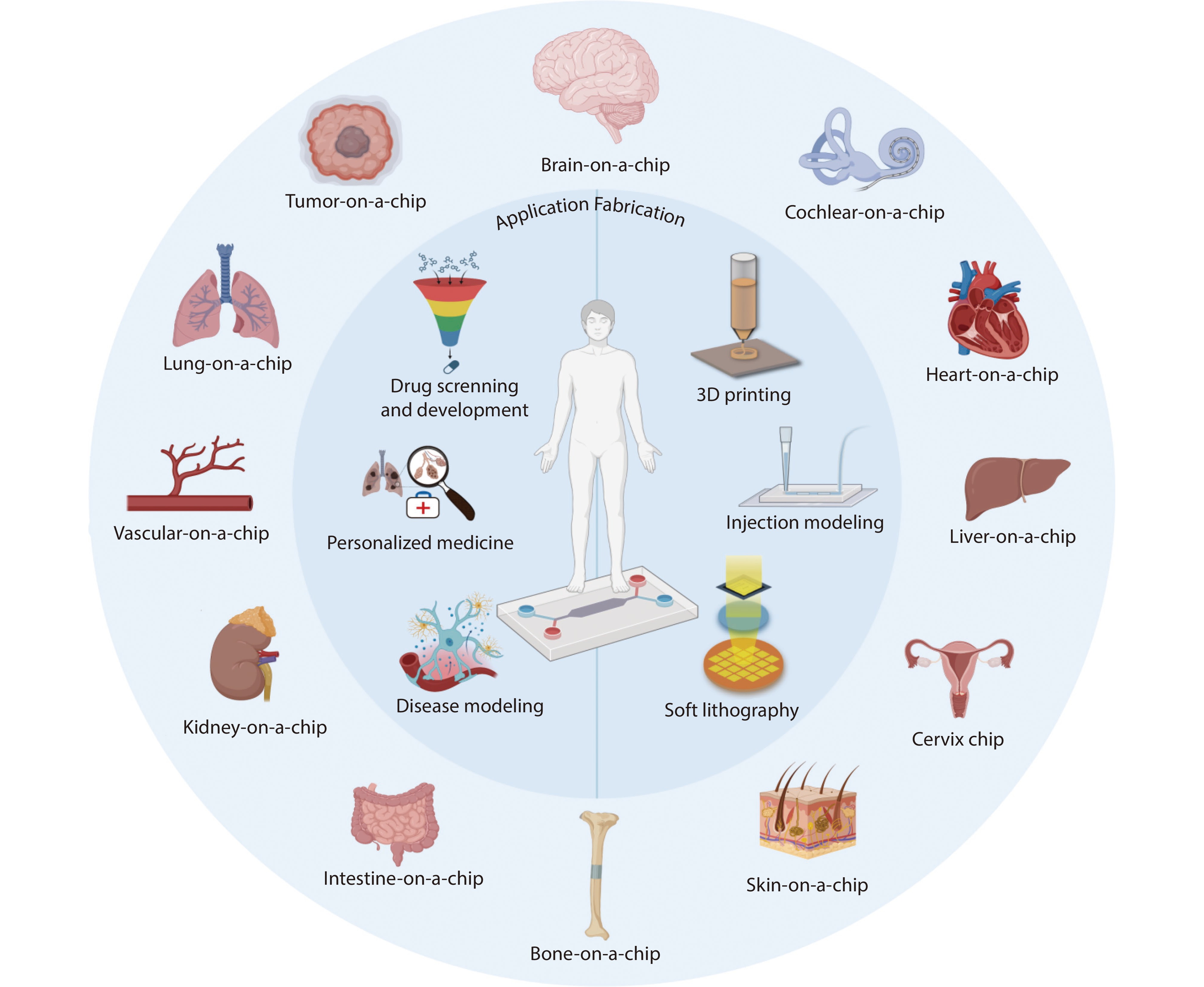
Citation: | Xinyue Han, Yuyang Qiu, Bin Zhang, Yunzhu Huang, Yusong Wang, Pan Feng, Xin Gao, Qian Zhu, Menghui Liao, Yangnan Hu, Renjie Chai. Advances in Organ-on-a-chip Technologies for Biomedical Research and Applications[J]. Materials Lab, 2025, 4(1): 240006. doi: 10.54227/mlab.20240006 |
Recent breakthroughs in micro-nano fabrication and tissue engineering have accelerated the evolution of organoids and organ-on-chips (OOCs), offering a novel
In recent years, advancements in micro-nanofabrication technologies and tissue engineering have propelled the development of organoids and organ-on-chips technologies, emerging as a promising approach for in vitro modeling of human organ physiology. Organ-on-chip devices utilize microfluidic systems to mimic the physiological microenvironment of specific organs, offering dynamic and flexible configurations to simulate a comprehensive human biological environment[1]. Applications in biomedicine include, but are not limited to, drug discovery, drug screening, and disease modeling. Normally, New drugs must go through a long process that typically takes around 10-15 years, from initial development to market availability. From the outset of the discovery process, through preclinical trials, into clinical studies, and ultimately securing regulatory approval, it is imperative to ensure the safety of human subjects in clinical trials[2]. Utilizing model organisms for fundamental research is a widely and readily adopted approach[3]. Nevertheless, in certain cases, although animal models have validated many drugs' efficacy, safety, and toxicity, the results in clinical III trials still fail to achieve the expected results[4], mainly due to the heterogeneity between human and animal models. This heterogeneity includes physiological differences, genetic differences and metabolic differences etc. For instance, regarding traumatic brain injury, individual animal models generally replicate only specific aspects, focusing on the pivotal injury mechanisms or a certain important pathophysiological course, which may fail to precisely reflect the complex, multi-factorial nature of severe head injuries seen in patients[5]. To address this issue, humanized mice and mouse-human chimeras have been developed. However, a key limitation remains in the genetic modification of these models—specifically, the challenge of achieving proper expression of human-specific cytokine transgenes during genetic alteration[6]. Meanwhile, there exists fundamental difference between animal models and humans, that their underlying molecular and cellular mechanisms are different[7], in such cases, in vitro testing in culture dishes may provide a more reliable and practical solution. Two-dimensional (2D) monolayer culture is less costly, faster, and more reproducible than animal models; however, this approach places cells in an unnatural environment without extracellular matrix (ECM)[8]. Three-dimensional (3D) cell culture enhances this model by more closely mimicking real-world conditions; It includes spheroid models, scaffold-dependent 3D cell cultures[9], and the derived organoids, organ-on-chips (OOCs), and organoids-on-chips, which will continue to play an essential role in future research (Figure 1).
The construction of diverse organoid models facilitates a better understanding of disease progression and organ function[10]. The fabrication of human tissues on chips aids in measuring tissue activity and functionality in a reproducible and high-throughput manner[11]. Currently, various disease models have been established, such as those for Alzheimer's disease[12], various tumor models[13], and the blood-brain barrier (BBB) model[14]. These in vitro models help address limitations found in animal models and other in vitro platforms that they cannot fully replicate and control their functions. Moreover, when evaluating the efficacy and toxicity of new drug candidates, traditional models often have low translational value. OOC technology has therefore garnered significant attention as an alternative platform for drug development and screening, leading to substantial advancements in global research[15]. OOCs refer to an organ micro-physiological system constructed on a slide-sized chip containing key elements of the organ microenvironment, for instance, living cells, tissue interfaces, biofluids and mechanical forces. Researchers design appropriate cytokines, growth factors, nutrients, ECM components, and connexins to mimic the desired tissues[16]. Emerging OOCs technology include brain-on-a-chip[17], cochlear-on-a-chip[18], vascular-on-a-chip[19], cervix chip[20], tumor-on-a-chip[21], intestine-on-chip[22], heart-on-a-chip[23], lung-on-a-chip[24], kidney-on-a-chip[25], bone on-chip[26], liver organ-on-chip[27], skin-on-a-chip[28], blood-brain barrier chips[29], multi-organ chip[30]. The fabrication of OOCs consists of two main aspects: biological modeling and chip design. The basis of biological modeling is selecting a suitable culture model to simulate the microenvironment of the human body. Previously, we discussed the limitations of the 2D culture model, which cannot adequately replicate the fluid-flow environment and the shear stresses exerted on cells. While microfluidic devices can address these needs, more complex models—such as those requiring reliable nutrient supply and enhanced cellular interactions—necessitate the use of 3D models. In chip design, the most commonly used microfluidic chips are made from polydimethylsiloxane (PDMS), which serve as soft materials. Utilizing this soft material, techniques such as 3D printing, injection molding or soft lithography technology[31] are employed to create individual compartments and microfluidic channels. Within these channels, living cells and tissues are arranged under dynamic fluid conditions, effectively simulating the structural and functional complexity of human tissues and organ units, as opposed to traditional cell culture methods[7].
In this review, we first introduce the strengths and weaknesses of various vitro drug screening and disease modeling platforms followed by an examination of how organ-on-chip models can effectively control the microenvironment (Figure 2). After constructing a highly biomimetic internal microenvironment, we analyzed the interaction patterns within the organ-on-chip system, determining whether it simulate tissue-tissue or multi-organ interactions. Finally, based on the above elaboration, we introduce the main application areas of OOCs and evaluate the technology's potential and future prospects.
2D cell culture is the most commonly used method in laboratories for culturing and maintaining cells. In this approach, cells typically adhere as a monolayer to a flat surface, such as in culture flasks and petri dishes, with a culture medium providing the necessary nutrients.[32]. 2D cell culture has long been used as a traditional in vitro model to study cellular responses to biophysical and biochemical signaling stimuli[33]. While it remains widely accepted and can provide insights into many biological activities and in vivo behaviors, it is evident that this approach has significant limitations. Monolayer cultures make it difficult to simulate the microenvironments in vivo and cellular structures[34], such as complex 3D tissue structures and cell-cell or cell-ECM interactions[35] which skew cellular bioactivities markedly away from in vivo responses. Additionally, it is challenging to simulate the complexity of human tumors accurately using 2D cell culture systems in cancer research, while the establishment of in vitro tumor models plays a crucial role in cancer therapy and the discovery of anticancer drugs. Conventional 2D tumor models are mainly limited to studying tumor progression and chemosensitivity[36]. Therefore, increasing attention has been focused on 3D cultures as an alternative, such as using various scaffolds like polymers or hydrogels for modeling and studying cancers in lung, breast, pancreatic, ovarian, bowel, and glioblastoma[37]. In the construction and utilization of 2D cell culture, it is also worth noting that surface design can play a targeted regulatory role. Researchers can regulate cell adhesion, growth, and differentiation by incorporating various types of factors, such as different materials, geometry, or types of biomolecules, and then modulate cell-substrate interactions by adjusting these variables, allowing for surface design in 2D cell culture that induces specific cellular responses. This approach aids in the deeper investigation of disease-related cell behaviors.[38].
In natural state, cells exist within the extracellular matrix (ECM). Three-dimensional (3D) cell culture, by constructing scaffolds to simulate the environmental structure of cells in living tissue, offers numerous advantages as a reliable alternative to 2D cell culture. Firstly, compared to 2D cell culture, synthetic ECM simulates physiological conditions and natural microenvironments ideally, such as structure, physiology[39], nutrients and diffusion of oxygen[40], usually provides complete cell-cell and cell-ECM interactions[41], which better promote cell attachment and migration. In contrast to animal models, data obtained in genetic analyses correlate better with human tissues[42]. Also, due to the biological differences between humans and animals, drug side effects that may go undetected in animal models can be identified directly by 3D cell culture without ethical concerns and regulatory issues associated with animal research[43].
However, in 3D cell culture, these scaffolds must meet certain conditions to achieve these functions. Firstly, the design of such scaffolds must have the required mechanical strength, appropriate pore size, porosity and mechanical properties within the tissue, and a certain degree of compatibility with the host[44]. From the perspective of scaffold materials, biomaterials that are highly similar to ECM are required to enhance cellular functions[45]. Various biopolymers are widely used in biomedical applications because of their distinctive characteristics. Natural biopolymers have lower toxicity, better bioactivity and biocompatibility[46], guiding the migration and growth of cells[47], but with poorer stability and processing properties. Synthetic polymers have better control over chemical composition in terms of processability and good mechanical properties. Hydrogels are widely used as a common polymer in tissue engineering due to their water-retaining property, softness and viscosity similarity to living tissues[48]. As early as the 1980s, some researchers found that laminin-rich gels could be used as a basement membrane to differentiate and generate mammary epithelial cells[49]. Three primary gels can be used as scaffolds: simple gels composed of collagen, ECM material extracted from relevant tissues to make their gels, and commercially available Matrigel[50], which exhibit great potential in tissue scaffolds, therapeutic delivery and 3D cell culture.
Organoids are another class of 3D self-assembled structures cultured[51]. Currently, organoids have been developed for the brain[52], retina[53], skin[54], heart[55], kidney[56], liver[57], intestine[58] and tumor[59]. Clinically, these organoids directly impact drug screening and personalized medicine, and also contributed to the foundational science of disease modeling[51]. Organoids, as a powerful in vitro system derived from adult stem cells (ASCs) or induced pluripotent stem cells (iPSCs), are 3D miniature in vitro organoid models capable of self-organizing and differentiating into organoids with structural and functional characteristics that are highly similar to the source tissues in terms of histology, cell type, and cell-specific function. By supplementing the culture medium with a specific mixture of tissue-specific growth factors, multiple kinds of organoids can be differentiated. In 2024, the Center for Drug Evaluation of the National Medical Products Administration in China issued the "Technical Guidelines for Nonclinical Research of Human Stem Cell Products," which explicitly stated that organoid models could be utilized as alternative models in nonclinical research. However, the construction of complex organoids encounters several challenges: vascularization, immunization, and systematization. One of the main restrictions of current organoid models is the absence of functional vascular compartments. Thus, the physiological relevance of organoid systems has not yet met the standards of human tissue construction[60]. Nevertheless, the emergence of organoids has presented new opportunities for tumor immunotherapy. Patient-derived tumor organoids have become a promising model for predicting personalized drug responses in cancer treatment, but they often lack immune components. Therefore, it is crucial to maintain the in vivo interaction between tumor cells and endogenous immune cells[61]. The onset of many diseases results from the combined action of multiple organ systems. While traditional single organoids can simulate the environment of a single organ in the body more realistically, they still have certain limitations for diseases that involve multiple systems. Thus, the construction of organoids that coordinate the function of various systems has become the ideal solution to address systemic issues.
Animal models with complex and complete physiological systems are the most common and effective in vivo test models to replace human subjects in clinical, pharmaceutical and scientific research and have already been used in biomedical research for thousands of years[62]. It has become the experimental model of choice in various studies, such as in the screening of anti-inflammatory drugs, where the assessment of plant constituents using animal models has proved to be the mainstay and backbone of specific anti-inflammatory drug development programs[63]. Rats and mice are the leading animal models widely used because of their high reproduction rate, ease of farming, and high homology to the human genome. However, they differ from humans in physiology and metabolism, which may affect the direct translation of drugs and the accuracy of disease modeling. Zebrafish, with its brain, digestive tract, muscle tissue, vascular system and innate immune system, is physiologically and genetically similar to humans and is equally easy to manipulate genetically. Moreover, their embryos are transparent and easy to observe, and they often have the same targets as humans for certain active drugs. It is also frequently used as an animal model in research because its heart is visible, and liver disease is similar to human disease[64]. The Drosophila melanogaster has 60% DNA sequence consistent with human’s regarding the genome. Still, it cannot simulate complex organs and systems, and it is challenging to accomplish complex behavioral cognition and immune system-related diseases[65]. In addition to the above standard animal models, large animals, including pigs[66], non-human primates (e.g., marmosets, rhesus monkeys, etc.)[67], dogs[68], and small and medium-sized animals such as rabbits[69] and guinea pigs[70] also have irreplaceable positions in the study of certain diseases. For example, the pig's anatomical, physiological, immunological, and genomic similarities to humans enhance its potential as a human model. Non-primates are the only possible model for in vivo studies of specific viruses such as HIV-1[71]. However, findings from animal studies cannot be simply applied to human studies[72]; for example, a distinct subpopulation of T cells, γδ T cells, does not develop identically in humans and mice[73]. Animals and humans belong to different strains. Animal models do not fully mimic the course of disease and drug action in the human body and have uncertain relevance to human clinical conditions[74], additionally, they may face high experimental costs and ethical issues[75], therefore, animal models may be unsuitable for certain clinical problem analysis.
OOCs are microfabrication devices developed on microfluidic chips to facilitate cell culture and emulate human organs, tissues, or microenvironments[76], offering a promising alternative for drug development. Organoids and OOCs share significant similarities; however, as traditional 3D culture models, organoids, while capable of constructing microtissue models, are still unable to fully replicate the essential characteristics of functional living organs, particularly in recreating complex microenvironments[77]. Therefore, by integrating technologies such as genome editing, 3D printing, advanced cell sensors, micro-nano fabrication, and microfluidics[78] , it becomes possible to truly construct intricate physiological structures (e.g., osteochondral/joint, epidermis, cochlea), fluid-producing and transporting structures (e.g., blood vessels, lymphatic vessels), and barrier structures (e.g., retina)[79] , allowing for precise control over spatial, temporal, and mechanical cues.
In organoid chips, controlling biochemical signals is one of the critical factors in simulating organ functions. Appropriate biochemical microenvironment is essential in inducing stem cells to generate organoids and maintain organoid homeostasis. Biochemical stimulation of various endogenous and exogenous signals is crucial[80]. Most organoids derive from an initial cell population exposed to a specific morphogen at a particular time, activating the desired developmental signaling pathway. In turn, self-organization can be triggered by adding these signals during organogenesis[81]. Of course, the additives required to prepare different organoids (such as cytokines, growth factors, and small molecules) are different, even for tissues and organs with very similar structures. At the same time, the concentration of additives can also affect organoid development. Chen et al. developed a tumor-metastasis-mimicked transwell-integrated organoids-on-a-chip platform (TOP). To simulate the behavior of tumor cells in obtaining nutrients from the surrounding environment, cytokine concentration gradients were established in the transwell and receiver chambers at the TOP. By measuring cytokine concentrations, a consistent and stable cytokine concentration gradient was observed between the transwell chamber and the receiving chamber (Figure 3a)[82]. Wiedenmann et al. designed a microporous chip to facilitate the differentiation of human induced pluripotent stem cells (hiPSC) into pancreatic duct-like organoids (PDLO). At different stages of hiPSC development, different supplements were added to the medium to induce directed differentiation of hiPSC. Bright-field images showed that pancreatic progenitor cells underwent morphological changes and eventually formed two different forms of PDLO. Immunofluorescence results showed that differentiated PDLO expressed catheter protein markers[83]. Tao et al. developed a microfluidic multi-organoid system. Liver and islet organoids were generated from hiPSC in polydimethylsiloxane (PDMS) microporous chips by adding different growth factors or small molecules to identified media at different stages of differentiation and were 3D co-cultured for up to 30 days. Co-cultured liver and islet organoids showed good growth and improved tissue-specific function (Figure 3b)[84]. Quilez et al. investigated the effect of morphogenetic hormone concentrations on spatial differentiation of skin organoids derived from pluripotent stem cells (PSO). By creating morphogenetic gradients in microfluidic spindle devices, it was found that different concentrations of BMP4 and LDN can spatially control dermal and epidermal differentiation in PSO, highlighting the complex balance of BMP4 and LDN concentrations in regulating this process (Figure 3c)[85]. The type and concentration of biochemical signals, the stage of biochemical signal addition, and the combination of different factors play a vital role in regulating the growth and differentiation of organoids in the chip.
Physical boundaries play a crucial role in cell and tissue organization during the development and homeostasis of organs in the body. Therefore, morphological guidance is an essential aspect of organ function simulation in organ chips, which affects the behavior of cells, the formation of tissues and the performance of organ functions. Through precise morphological guidance, such as 3D printing, microfluidic channel design, extracellular matrix simulation, micropattern technology, mechanical force application and intercellular communication regulation, the three-dimensional structure and function of human organs can be simulated to achieve a high degree of control over cell growth and tissue morphology[86]. These technologies have been applied in organ-on-a-chip models such as intestines, lungs, hearts and tumors[87], which not only help to understand the physiological and pathological processes of organs but also provide a powerful experimental platform for drug screening, disease simulation and tissue engineering[88].
For example, Elci et al. produced a hydrogel-on-a-chip organoid model for developing functional tubular biliary organoids. To increase the complexity at tissue-level, a perfusable branching network is formed by employing a preset geometry that accurately replicates the intrahepatic biliary tree’s complicated structure. The resultant constructs exhibit long-term stability while maintaining their identity as biliary organoids, showcasing essential cholangiocyte features such as transport activities and tubular morphology with apicobasal polarity (Figure 4a)[89]. Quilez et al. propose a novel culture method using a 3D bio-printed spindle-shaped hydrogel device to generate PSOs in a chemically defined 3D ECM environment. The numerical simulation results show that the anoxic region in spindle PSO is smaller than that in spherical PSO. Meanwhile, immunofluorescence results of Caspase 3, an early apoptotic marker, also showed that PSO generation in 3D ECM with spindle geometry could alleviate necrosis (Figure 4b)[85]. Mitrofanova et al. have developed an advanced human colon organoid, the "mini colon," which simulates the human colon's physiological function and pathological state by integrating organoid and organ-on-a-chip technology. The research team employed a combination of microprocessing techniques, hydrogel engineering, and the intrinsic self-organizing capabilities of stem cells to direct mouse intestinal stem cells in forming perfusable, tubular tissues. These engineered tissues exhibited crypt and villus domains that closely resemble those found in the native intestine (Figure 4c)[90]. At the same time, Gjorevski et al. developed a new approach to forming more deterministic intestinal organoids through spatial and temporal control by investigating how tissue geometry drives deterministic organoid pattern formation. The team used photosensitive hydrogel techniques and soft lithography to specify the initial geometry of the gut organoids, thereby controlling their patterns and crypt formation. Through optical modeling technology, the formation of crypts of organoids can be controlled in a predetermined area, and high-fidelity spatial control is realized. At the same time, by adjusting the initial geometry of organoids, the formation position of crypts can be predicted and controlled (Figure 4d)[91]. Jian et al. fabricated HepaRG/HUVECs/LX-2 loaded liver organoids with bionic lobular structures through a bioprinting strategy based on multicellular 3D droplets. After 7 days of culture, liver organoids are able to preserve structural integrity and multicellular distribution within printed lobular structures. At the same time, cell proliferation results measured by absorbance at 450 nm showed that cells embedded in liver organoids showed higher cell viability within 7 days compared to cells cultured in 2D monolayer (Figure 4e)[92]. This model not only shows cell diversity and maturity similar to that of the human colon but also maintains a steady state culture for a long time and accurately simulates the impact of drugs on the intestine, providing an innovative experimental platform for studying intestinal physiology and pathology, and showing great potential in drug safety assessment.
Microfluidic-based organ-on-a-chip technology has the inherent advantage of being able to simulate the shear forces from fluid perfusion. Precise control of parameters such as flow rate, direction, frequency, and flow pattern can enable various functions and applications. Shear stress alterations in arteries are closely linked to the onset and progression of vascular diseases, for instance, coronary artery disease and abdominal aortic aneurysm (AAA). Paloschi et al. developed an Artery-on-a-Chip (AoC) which simulates the structure and function of medium to large arteries to explore the pathogenesis behind these diseases. Using an external microfluidic pressure controller and precise flow rate regulation, AoC can apply a constant shear stress on the endothelial cell layer, simulating the effects of blood flow on the arterial wall in vivo (Figure 5a)[93]. In addition, by simulating the conditions of intertissue flow, the shear damage to cells can be reduced. For example, the dynamic culture of islet cell spheroids in Jun et al., compared with static culture, can enhance the health of pancreatic islets and the maintenance of endothelial cells (Figure 5b)[94]. Squamous cell carcinoma, one of the most common forms of cervical cancer, can be roughly divided into exophytic, endophytic, ulcerative, and cervical gland types based on its growth characteristics and macroscopic morphology. Controlling the flow frequency of shear forces can promote different expression types of cells. For example, Izadifar et al. described a human cervical mucosal organ chip model, which simulated in vivo shear forces by controlling continuous or intermittent flow conditions. The results indicated that the shear troops significantly influenced the differentiation of cervical epithelial cells, mucus composition, and immune response. At the same time, cells cultured under continuous flow conditions tend to express an exocervical phenotype. In contrast, those cultured under intermittent flow conditions are more inclined to express an endocervical phenotype (Figure 5c)[20]. It is well known that intestinal peristalsis is an essential part of intestinal function, which contributes to the digestion and absorption of food. It also affects the distribution and activity of bacteria in the gut, and alternations in the gut microflora can further impact intestinal peristalsis[95]. Grassart et al. also demonstrated that intestinal peristalsis can provide a favorable adhesion and invasive environment for bacteria in the crypts by simulating the flow of solid fluids and peristalsis in the intestinal tract[96]. The infection efficiency of Shigella flexneri was found to increase significantly under flow conditions, and the invasive ability of the bacteria was found to decrease significantly when the flow rate was accelerated. In fact, fluid flow alone had no significant effect on the infected area. While the stretching forces that replicate intestinal peristalsis significantly expand the area of infection. Moreover, the combination of the two mechanical forces appears to amplify both the area of infection and the bacterial colonization capacity. In short, fluid shear stress, as an external force, primarily affects tissues and organs such as arteries and intestines that function under peristaltic conditions. The flow conditions better mimic the original in vivo state, allowing for the observation and replication of results in vitro.
Intrinsic mechanical forces (mechanical tensile and compressive forces) and extrinsic mechanical forces (fluid shear generated around the cell layer) guide early embryonic development[97]. Subsequently, during later embryonic stages, the formation of almost all organs is sensitive to the mechanical cues from their surroundings[98], which are intimately linked to the cell-ECM interactions[97]. These interactions generate a spectrum of mechanical forces that are crucial for shaping various tissues such as the brain, lungs, kidneys, muscles, blood vessels, mammary glands, and cartilage, as well as for bone formation[98], and the role that mechanical forces play cannot be ignored in the development of related organoids. Therefore, the influence of mechanical forces needs to be carefully considered in developing organ-on-a-chip technologies, especially in lung and heart chip designs, where intrinsic self-forming forces are fundamental and must be present. For example, during the design of a lung chip, a color-indicative biomimetic alveolar system with vivid mechanical responses was created, co-culturing type II alveolar cells (ATII) and vascular endothelial cells (ECs) to rebuild the alveolar-capillary barrier in vitro (Figure 6a)[99]. The internal inverse opal structured polyurethane (IOS-PU) membrane with ultra-high elasticity can simulate the contraction and expansion of alveoli. By changing the gas infusion volume in the chamber, the stretching degree of the membrane increases from 10% to 79%, and the IOS-PU membrane can gradually change from yellow-green to blue. This membrane has been proven to have good biocompatibility and can be widely used as a naked-eye pull-depth sensor in simulating dynamic mechanical changes. Xu et al. also applied this inverse opal structure to develop a human heart chip system. The anisotropic structural color hydrogel integrated into the organ chip device provides a spatiotemporally uniform fluid environment for human induced pluripotent stem cell-derived cardiomyocytes (hiPSC-CMs) in multichannel culture microfluidic chambers, for real-time and visual environmental cardiac toxicity screening (Figure 6b)[100]. The spontaneous beating of hiPSC-CMs involves contraction movements that induce the bending of the living anisotropic hydrogel, further leading to structural color changes. Zhu et al. also established a novel biomimetic 3D microphysiological lung chip system with visual breathing, which can simulate the breathing process of alveoli cyclically rather than a simple unidirectional cyclic stretching (Figure 6c)[101]. The PDMS film is used as the substrate, with its surface coated in structural color materials; These materials can visually display cellular mechanics and pathological changes through changes in structural color. Low and high airflow cycles simulate the expansion and contraction of alveoli during the breathing process by inducing the deformation of the PDMS film with periodic airflow. In general, the effects of this internal tension and compression force are not easy to visualize, and the studies described above are all through the use of new materials to solve this problem. In addition, micro-sensors, such as pressure sensors, can be integrated into the organ chip to monitor and record the mechanical changes within the chip in real time[102].
The tissue-tissue and multiorgan interactions model both focus on simulating the interactions and communication between different tissues or organs in the body. However, the tissue-tissue interaction model is more focused on the interactions between two specific tissues or organs, with a clear target and easier to implement and build, while the multiorgan model simulates the complex interactions between multiple organs.In both physiological development and in vitro experimentation, maintaining interactions between different tissue types is crucial for organogenesis and homeostasis. In vitro studies create a microenvironment that mimics in vivo conditions and facilitates tissue-tissue interactions, rather than replicating intact organs, offers significant advantages for research. For instance, a model featuring retinal organoids (ROs) with retinal pigment epithelium (RPE) demonstrates this approach[103]. While retinal organoids typically contain RPE, their arrangement differs from in vivo, with RPE forming clusters alongside photoreceptors[104]. In this model, human induced pluripotent stem cell (hiPSC)-derived RPE cells and ROs embedded in hyaluronic acid-based hydrogels are sequentially injected into two top-layer tissue chambers using a microfluidic platform. At the same time, the bottom layer simulates the vasculature to supply nutrients and compounds. This model enhances the potential for drug development and offers novel avenues for studying retina-related diseases. However, if not properly controlled during in vitro culture—especially with stem cell derived tissues—the shape and size of the organs can become unpredictable under permissive conditions. Therefore, to better simulate these complex tissue-tissue interactions, the organoids can be spatially pre-patterned during the initial design of the organoids to make them more similar to the function and complexity of real organs[105] by arranging the different organoids or cell types within the chip according to specific spatial locations.
Current tissue-tissue interaction models and single-organ models are insufficient for replicating the intricate interactions among different organs or tissues in vivo, this is because there is a lack of comprehensive understanding of the interplay between multiple organ systems, making it difficult to extrapolate to the entire body. However, maintaining multiple organ interactions and achieving homeostasis is crucial in addressing diseases with complex pathologies. A strategy to address this challenge is employing two organ chip co-culture designs. Various multi-organ chips, such as pancreas-liver (Figure 7a)[106] kidney-liver (Figure 7b)[107], pancreatic islets-liver spheroids[108], lung-liver[109],intestinal-liver models[110], bone-heart-liver-vascular (Figure 7c)[111], and liver-heart-bone-skin[30] gut-immune-skin (Figure 7d)[112] are currently available. Body-on-a-chip (BOC) systems are multi-organ systems, which can also be referred to multi-organ microphysiological systems (MOM) and multi-organ-on-a-chip (MOC)[113]. To comprehensively investigate the impact of orally administered nanoparticles on liver tissues, a “gastrointestinal tract-liver-other tissues” BOC system model was developed[114]. This model integrated an in vitro setup comprising human intestinal cells Caco-2 and mucin-producing HT29-MTX cells with an in vitro representation of the liver using HepG2/C3A cells within a microfluidic device. Combining MTX cells and HepG2/C3A cells in a microfluidic platform established two fluidic circuits: a vessel housing carboxylated polystyrene nanoparticles with a gastrointestinal model circuit and a human circulatory circuit. This dual-organ system facilitated the observation of both inter-liver tissue interactions and the compounded effects of interactions between the gastrointestinal tract and the liver. Additionally, Vivian et al.[107] integrated human kidney and liver-like organs using microfluidic channels, establishing a kidney injury model employing hydrogen peroxide. Liver-like organs and 2D-cultured renal tubules were inserted into each of the two compartments to be co-cultured in the TissUse HUMIMIC Chip 2 microfluidic model (TissUse GmbH), which enabled the study of renal-hepatic interactions in dynamic MOC culture. Another strategy involves developing a sophisticated microarray metastasis system. Cancer metastasis is a complex pathophysiological phenomenon that disseminates cancer cells from the primary site to distant organs[115]. The advancement of tumor microarray models, encompassing multi-organ interactions, is still in its early stages[116]. Currently, the organ microarray model of cancer metastasis primarily encompasses a tumor growth and invasion model, angiogenesis model, lymphatic system model, endocytosis model, exocytosis model, organ-specific metastasis model, and tumor stroma model[117]. This comprehensive model effectively captures nearly every aspect of the metastatic cascade, thus offering valuable insights into cancer progression and treatment strategies.
OOCs have a wide range of applications in medical research, drug development, and disease treatment. This section provides a detailed introduction to three applications, including drug screening and development, disease modeling and personalized medicine. Taking Zou et al.'s study as an example[118], in this research, a co-culture system of patient-derived organoid (PDO) and mesenchymal stem cells (MSC) was utilized to simulate the native tumor microenvironment (TME) for predicting the efficacy of anti-hepatocellular carcinoma (HCC) immunotherapies. The study provided personalized treatment plans based on individual patient's tumor characteristics and modeled the original tumor model. In addition, PDO was cultured on a chip, and various drugs, including chemotherapeutic agents, targeted therapies, and immunotherapeutic drugs, were tested to assess their impact on tumor growth. This approach enables the rapid and efficient screening of potentially effective drugs for specific patients. Therefore, the study offers a new platform for personalized medicine and aids in accelerating the development of new therapies through disease modeling and drug screening, providing patients with more effective treatment options. In the following section, we will detail three OOC applications.
The high failure rate of new drug development in clinical trials is primarily due to our insufficient understanding of the basic pathophysiology of the human body and its underlying mechanisms. The drug discovery process can be conceptually divided into target selection, lead compound identification, and preclinical research[22]. OOCs can precisely locate drug targets in a controllable and traceable manner within simulated human systems[119]. For instance, intestinal ischemia/reperfusion (IR) injury, which has a mortality rate of 50%-80%, is a typical cascade injury disease that ultimately triggers inflammation and destroys epithelial cells. Therefore, it is imperative to identify drug targets to alleviate epithelial damage at the source[120]. This study developed an updated intestinal organ-on-a-chip, and with the support of this technology, combined with RNA sequencing results, it was found that Olfm4 is the most significantly down-regulated gene in intestinal organoids after rapid hypoxia followed by oxygenation (HR) treatment. The OLFM4 protein encoded by this gene can inhibit the activation of the NF-kappa B signaling pathway, lowering intestinal inflammation, thus making it a therapeutic target. During the lead optimization phase, by incorporating OOC research into this stage of drug development compounds that produce toxic signals in human organ chips can be eliminated early in vivo studies. This can reduce animal testing and ensure safer drug candidates for the subsequent development process. Moreover, it is important to understand how multiple organs and tissues respond to specific drugs. Administration routes that have been simulated include oral/rectal administration through intestinal chips, inhalation administration through lung chips, intravenous administration through vascularized models, and transdermal administration through skin chips[88]. For instance, Zhu et al. constructed a dynamic Microphysiological System Chip Platform (MSCP) that includes intestinal, liver, heart, and lung cancer spheroid cells[121]. This MSCP is capable of simultaneously culturing 304 spheroids and features four parallel drug injection channels. In their study, A549 lung cancer cell spheroids were cultured on the MSCP to evaluate the potency of four chemotherapeutic drugs: cisplatin, docetaxel, pemetrexed, and doxorubicin. Ultimately, it was concluded that doxorubicin exhibited the most robust efficacy against A549 cell spheroids, while pemetrexed showed the weakest (Figure 8a).
The screening and development of drugs is a lengthy process. Identifying the correct research direction and selecting the most suitable research methods can significantly shorten this duration. Therefore, choosing appropriate disease models in the early stages of drug discovery is crucial. For some general studies, researchers often default to use animal models. However, for the study of complex diseases or when there are significant differences between human and animal models of a particular disease, animal models are no longer the optimal choice. For example, modeling lung damage and immune responses caused by the SARS-CoV-2 virus to accelerate research and drug development for COVID-19 (Figure 8b)[122], and modeling the different inflammatory responses of type II diabetes patients to COVID-19[123] or research related to cardiovascular diseases involves limitations in the translational ability of human diseases and the limitations in replicating human natural cardiac physiology and drug responses[124]. The introduction of OOCs can properly solve this problem. As an in vitro model, it owns the unique advantages of high throughput, repeatability and the ability to simulate human physiological states adequately. Most importantly, the OOCs model can almost simulate any tissue, organ, cytomatrix, and their interactions in vitro that researchers want to analyze. It is thus regarded as favorable option for disease model. Currently, various disease models have been established using the OOCs model, such as a human aging research model[125], Parkinson's disease (PD), Alzheimer's disease (AD)[126], cancer models[127], and inflammatory models interacting with the human immune system[128]. However, the research on OOCs is still in its infancy, and issues such as how to achieve the overall interaction of multi-organ systems still limit the application of OOC models in disease modeling. However, the contribution of this model in improving the understanding, diagnosis, prevention, and treatment of diseases is prominent.
Personalized medicine is a medical model that provides customized treatment plans for each patient based on individual biological information, lifestyle, and environmental factors. It aims to maximize treatment efficacy and minimize new and existing drugs' side effects and costs through more precise diagnosis and treatment. It not only achieves treatment but also prevention, thereby improving the quality of life for patients[16]. For example, Ge et al. provided customized drug delivery schemes for cancer patients, facilitating more effective and safer treatments[129]. In this study, through microfluidic chip technology, they captured and purified patient-derived tumor extracellular vesicles (PT-EVs) from patient-derived tumor models (PDMs) (Figure 8c). They loaded two commonly used drugs for the clinical treatment of hepatocellular carcinoma (HCC), Lenvatinib and doxorubicin (DOX), into PT-EVs. Utilizing the natural targeting ability of PT-EVs, they precisely delivered the drugs to matching in vitro tumor models for drug sensitivity testing. Personalized medicine relies on advanced technology and large-scale data analysis. Existing high-throughput, data-intensive biomedical testing methods include DNA sequencing, proteomics, medical imaging, bioinformatics, and data analysis, etc.[130]. Cells can be collected from individuals to generate induced pluripotent stem cells (iPSCs), which can produce corresponding organs and organoids for studying specific diseases in vitro[131]. Compared to animal models, it has become a better choice when “genetic humanization” is required or when there is a significant difference in physiology between the model and human physiology; iPSCs have successfully ushered unprecedented precision and a new era of personalized medicine[132]. As a reliable time and cost-saving drug screening platform, OOCs can be used as a new perspective of high-throughput solution for personalized drug development.
In recent years, breakthroughs in OOC technology have significantly accelerated drug development. As a cutting-edge approach for in vitro disease modeling, OOC technology holds the potential to substantially shorten drug evaluation cycles while enhancing accuracy. In 2015 OOC technology was recognized as a revolutionary methodological approach with the potential to replace animal testing in drug development and disease modeling[133]. In 2017, the Food and Drug Administration (FDA) reached a consensus with several chip suppliers to introduce OOC technology into laboratories, aiming to regulate the technology and respond to the FDA's efforts in the field of toxicology[134]. In 2021, the FDA's Center for Drug Evaluation and Research (CDER) assessed the reproducibility of 3D OOC models, seeking relevant data to establish quality control and performance standards for the OOC industry[135]. Furthermore, enterprises have integrated OOC technology with AI pharmaceutical techniques, developing end-to-end AI-driven innovative drug development systems. These systems aim to replace traditional laboratory practices with standardized and automated approaches, enabling users to construct desired organ microtissues in more straightforward and reproducible ways, thus significantly reducing application expense. Industry research organizations predict rapid growth in the global OOCs market, with a compound annual growth rate over 30% expected by 2030[136]. Additionally, the field of organ-on-a-chip has advanced significantly, with some models now achieving a higher level of simulation than animal models. It is expected to make breakthroughs in its large-scale application, especially in the development of new drugs.
However, OOCs also face numerous challenges, with high cost being a primary hindrance to widespread adoption. As an emerging technology, OOC research is still relatively limited and in its early stage of development. Completely replacing human organs with this technology is not yet feasible. Instead, current efforts focus on constructing characteristic interfaces that allow OOC to replicate key organ functionalities of interests. Furthermore, the research for OOCs lacks uniform evaluation standards, making selecting appropriate construction models a time-consuming and costly endeavor. While microfluidic technologies have increased OOC throughput, this has led to a surge in data generation, underscoring the importance of efficient data collection and analysis. Some studies have turned to robotics to operate chips and collect data, employing machine learning for data analysis[137]. The preferred material for OOCs, PDMS, may raise another research difficulties. PDMS has a high uptake of some small molecule materials, which can have an impact on the drug concentration over time during drug screening is performed. Therefore, selecting suitable materials for OOCs is also essential[138]. Additionally, the heterogeneity of non-autologous iPSCs and the derived phenotypic cells lack maturity, so how to develop disease models with apparent cellular features and homogeneous populations of target phenotypic cells needs to be further analyzed in future therapeutic applications[139]. In addition, as the technology develops, ethical issues deserve to be discussed. For example, organ on chips made from human organs are more specialized, complex and sensitive. Donors or patients have the right to know how their cells or tissues will be used and to obtain explicit consent. And, when some models have human characteristics, this may affect public acceptance of related research. Finally, we recognize that some OOC platforms have already been successfully commercialized. However, broader application in the pharmaceutical industry will require continuous technological improvements and cost reductions to bridge the gap between academia and industry[140].
Ethics approval and consent to participate
Not applicable.
Consent for publication
Not applicable.
Competing interests
The authors declare that they have no competing interests.
Not applicable.
RJC, YNH, MHL and QZ defined the review's focus. XYH and BZ summarized studies. XYH and YYQ drafted the manuscript. BZ and YZH participated in some parts of the final manuscript. YSW, PF and XG revised the manuscript. All authors reviewed the final version of the manuscript. All authors read and approved the final manuscript.
1. | Z. Li, D. Yu, C. Zhou, F. Wang, K. Lu, Y. Liu, J. Xu, L. Xuan, X. Wang, Biomater. Transl., 2024, 5, 21 |
2. | L. H. Chi, A. D. Burrows, R. L. Anderson, Drug Discov. Today, 2022, 27, 257 |
3. | E. V. Romanova, J. V. Sweedler, ACS Chem. Neurosci., 2018, 9, 1869 |
4. | M. R. Bullock, B. G. Lyeth, J. P. Muizelaar, Neurosurgery, 1999, 45, 207 |
5. | S. X. Li, B. W. Wang, D. Liu, G. L. He, H. Wang, Y. J. Duan, J. J. Xing, H. Y. Zhou, Y. W. Zhou, Fa Yi Xue Za Zhi, 2011, 27, 286, 294 |
6. | L. D. Shultz, F. Ishikawa, D. L. Greiner, Nat. Rev. Immunol., 2007, 7, 118 |
7. | D. E. Ingber, Nat. Rev. Genet., 2022, 23, 467 |
8. | E. Suarez-Martinez, I. Suazo-Sanchez, M. Celis-Romero, A. Carnero, Cell Biosci., 2022, 12, 39 |
9. | E. T. Verjans, J. Doijen, W. Luyten, B. Landuyt, L. Schoofs, J. Cell Physiol., 2018, 233, 2993 |
10. | S. Kheiri, I. Yakavets, J. Cruickshank, F. Ahmadi, H. K. Berman, D. W. Cescon, E. W. K. Young, E. Kumacheva, Adv Mater., 2024, 36, 2410547 |
11. | S. Ahadian, R. Civitarese, D. Bannerman, M. H. Mohammadi, R. Lu, E. Wang, L. Davenport-Huyer, B. Lai, B. Zhang, Y. Zhao, S. Mandla, A. Korolj, M. Radisic, Adv. Healthc. Mater., 2018, 7, 1700506 |
12. | F. M. Pramotton, S. Spitz, R. D. Kamm, Adv. Sci. (Weinh), 2024, 11, e2403892 |
13. | G. S. Offeddu, E. Cambria, S. E. Shelton, K. Haase, Z. Wan, L. Possenti, H. T. Nguyen, M. R. Gillrie, D. Hickman, C. G. Knutson, R. D. Kamm, Adv. Sci. (Weinh), 2024, 11, e2402757 |
14. | A. R. Mulay, J. Hwang, D. H. Kim, Adv. Healthc. Mater., 2024, 13, e2303180 |
15. | V. Carvalho, I. M. Gonçalves, N. Rodrigues, P. Sousa, V. Pinto, G. Minas, H. Kaji, S. R. Shin, R. O. Rodrigues, S. Teixeira, R. A. Lima, Comput. Methods Programs Biomed., 2024, 243, 107883 |
16. | N. Tabatabaei Rezaei, H. Kumar, H. Liu, S. S. Lee, S. S. Park, K. Kim, Adv. Healthc. Mater., 2023, 12, e2203172 |
17. | R. O. Rodrigues, S. R. Shin, M. Bañobre-López, J. Nanobiotechnology, 2024, 22, 573 |
18. | Y. Hu, J. Xing, H. Zhang, X. Pang, Y. Zhai, H. Cheng, D. Xu, M. Liao, Y. Qi, D. Wu, B. Zhang, L. Cheng, B. Chu, C. Zhang, Y. Zhao, R. Chai, Adv. Mater., 2024, 36, e2309002 |
19. | K. Ohashi, A. Hayashida, A. Nozawa, S. Ito, Curr. Res. Toxicol., 2024, 6, 100163 |
20. | Z. Izadifar, J. Cotton, S. Chen, V. Horvath, A. Stejskalova, A. Gulati, N. T. LoGrande, B. Budnik, S. Shahriar, E. R. Doherty, Y. Xie, T. To, S. E. Gilpin, A. M. Sesay, G. Goyal, C. B. Lebrilla, D. E. Ingber, Nat. Commun., 2024, 15, 4578 |
21. | K. E. de Roode, K. Hashemi, W. P. R. Verdurmen, R. Brock, Small, 2024, 20, e2402311 |
22. | O. T. P. Nguyen, P. M. Misun, A. Hierlemann, C. Lohasz, Adv. Healthc. Mater., 2024, 13, e2302454 |
23. | E. Jastrzebska, E. Tomecka, I. Jesion, Biosens. Bioelectron., 2016, 75, 67 |
24. | I. Francis, J. Shrestha, K. R. Paudel, P. M. Hansbro, M. E. Warkiani, S. C. Saha, Drug Discov. Today, 2022, 27, 2593 |
25. | K. T. Kroll, M. M. Mata, K. A. Homan, V. Micallef, A. Carpy, K. Hiratsuka, R. Morizane, A. Moisan, M. Gubler, A. C. Walz, E. Marrer-Berger, J. A. Lewis, Proc. Natl. Acad. Sci. USA, 2023, 120, e2305322120 |
26. | Y. Hu, H. Zhang, S. Wang, L. Cao, F. Zhou, Y. Jing, J. Su, Bioact. Mater., 2023, 25, 29 |
27. | T. Messelmani, L. Morisseau, Y. Sakai, C. Legallais, A. Le Goff, E. Leclerc, R. Jellali, Lab Chip, 2022, 22, 2423 |
28. | E. Sutterby, P. Thurgood, S. Baratchi, K. Khoshmanesh, E. Pirogova, Small, 2020, 16, e2002515 |
29. | G. D. Vatine, R. Barrile, M. J. Workman, S. Sances, B. K. Barriga, M. Rahnama, S. Barthakur, M. Kasendra, C. Lucchesi, J. Kerns, N. Wen, W. R. Spivia, Z. Chen, J. Van Eyk, C. N. Svendsen, Cell Stem Cell, 2019, 24, 995 |
30. | K. Ronaldson-Bouchard, D. Teles, K. Yeager, D. N. Tavakol, Y. Zhao, A. Chramiec, S. Tagore, M. Summers, S. Stylianos, M. Tamargo, B. M. Lee, S. P. Halligan, E. H. Abaci, Z. Guo, J. Jacków, A. Pappalardo, J. Shih, R. K. Soni, S. Sonar, C. German, A. M. Christiano, A. Califano, K. K. Hirschi, C. S. Chen, A. Przekwas, G. Vunjak-Novakovic, Nat. Biomed. Eng., 2022, 6, 351 |
31. | J. Ko, D. Park, S. Lee, B. Gumuscu, N. L. Jeon, Micromachines (Basel), 2022, 13, 1200 |
32. | S. Breslin, L. O'Driscoll, Drug Discov. Today, 2013, 18, 240 |
33. | K. Duval, H. Grover, L. H. Han, Y. Mou, A. F. Pegoraro, J. Fredberg, Z. Chen, Physiology (Bethesda), 2017, 32, 266 |
34. | B. D. Cardoso, E. M. S. Castanheira, S. Lanceros-Méndez, V. F. Cardoso, Adv. Healthc. Mater., 2023, 12, e2202936 |
35. | D. Lv, Z. Hu, L. Lu, H. Lu, X. Xu, Oncol Lett, 2017, 14, 6999 |
36. | V. L. Dsouza, R. Kuthethur, S. P. Kabekkodu, S. Chakrabarty, Biochim. Biophys. Acta Rev. Cancer, 2022, 1877, 188717 |
37. | W. H. Abuwatfa, W. G. Pitt, G. A. Husseini, J. Biomed. Sci., 2024, 31, 7 |
38. | M. H. Kim, M. Kino-oka, M. Taya, Biotechnol. Adv., 2010, 28, 7 |
39. | O. Habanjar, M. Diab-Assaf, F. Caldefie-Chezet, L. Delort, Int. J. Mol. Sci., 2021, 22, 12200 |
40. | C. Vila-Parrondo, C. García-Astrain, L. M. Liz-Marzán, Adv. Colloid Interface Sci., 2020, 283, 102237 |
41. | J. H. Ylostalo, Cells, 2020, 9, 2178 |
42. | V. Brancato, J. M. Oliveira, V. M. Correlo, R. L. Reis, S. C. Kundu, Biomaterials, 2020, 232, 119744 |
43. | S. Liu, S. Kumari, H. He, P. Mishra, B. N. Singh, D. Singh, S. Liu, P. Srivastava, C. Li, Biosens Bioelectron, 2023, 231, 115285 |
44. | A. Zielińska, J. Karczewski, P. Eder, T. Kolanowski, M. Szalata, K. Wielgus, M. Szalata, D. Kim, S. R. Shin, R. Słomski, E. B. Souto, J Control Release, 2023, 359, 207 |
45. | Y. Park, K. M. Huh, S. W. Kang, Int. J. Mol. Sci., 2021, 22, 2491 |
46. | W. L. Stoppel, C. E. Ghezzi, S. L. McNamara, L. D. Black, 3rd, D. L. Kaplan, Ann. Biomed. Eng., 2015, 43, 657 |
47. | P. B. Malafaya, G. A. Silva, R. L. Reis, Adv. Drug Deliv. Rev., 2007, 59, 207 |
48. | S. Van Vlierberghe, P. Dubruel, E. Schacht, Biomacromolecules, 2011, 12, 1387 |
49. | S. A. Yi, Y. Zhang, C. Rathnam, T. Pongkulapa, K. B. Lee, Adv. Mater., 2021, 33, e2007949 |
50. | A. Abbott, Nature, 2003, 424, 870 |
51. | T. Takahashi, Annu. Rev. Pharmacol. Toxicol., 2019, 59, 447 |
52. | C. A. Trujillo, A. R. Muotri, Trends Mol. Med., 2018, 24, 982 |
53. | C. S. Cowan, M. Renner, M. De Gennaro, B. Gross-Scherf, D. Goldblum, Y. Hou, M. Munz, T. M. Rodrigues, J. Krol, T. Szikra, R. Cuttat, A. Waldt, P. Papasaikas, R. Diggelmann, C. P. Patino-Alvarez, P. Galliker, S. E. Spirig, D. Pavlinic, N. Gerber-Hollbach, S. Schuierer, A. Srdanovic, M. Balogh, R. Panero, A. Kusnyerik, A. Szabo, M. B. Stadler, S. Orgül, S. Picelli, P. W. Hasler, A. Hierlemann, H. P. N. Scholl, G. Roma, F. Nigsch, B. Roska, Cell, 2020, 182, 1623 |
54. | J. Lee, W. H. van der Valk, S. A. Serdy, C. Deakin, J. Kim, A. P. Le, K. R. Koehler, Nat. Protoc., 2022, 17, 1266 |
55. | Y. R. Lewis-Israeli, A. H. Wasserman, M. A. Gabalski, B. D. Volmert, Y. Ming, K. A. Ball, W. Yang, J. Zou, G. Ni, N. Pajares, X. Chatzistavrou, W. Li, C. Zhou, A. Aguirre, Nat. Commun., 2021, 12, 5142 |
56. | K. A. Homan, N. Gupta, K. T. Kroll, D. B. Kolesky, M. Skylar-Scott, T. Miyoshi, D. Mau, M. T. Valerius, T. Ferrante, J. V. Bonventre, J. A. Lewis, R. Morizane, Nat. Methods, 2019, 16, 255 |
57. | S. J. Mun, J. S. Ryu, M. O. Lee, Y. S. Son, S. J. Oh, H. S. Cho, M. Y. Son, D. S. Kim, S. J. Kim, H. J. Yoo, H. J. Lee, J. Kim, C. R. Jung, K. S. Chung, M. J. Son, J. Hepatol., 2019, 71, 970 |
58. | S. Rahmani, N. M. Breyner, H. M. Su, E. F. Verdu, T. F. Didar, Biomaterials, 2019, 194, 195 |
59. | B. L. LeSavage, R. A. Suhar, N. Broguiere, M. P. Lutolf, S. C. Heilshorn, Nat. Mater., 2022, 21, 143 |
60. | N. Werschler, C. Quintard, S. Nguyen, J. Penninger, Atherosclerosis, 2024, 398, 118529 |
61. | S. C. Cordts, K. Yuki, M. F. Henao Echeverri, B. Narasimhan, C. J. Kuo, S. K. Y. Tang, Microsyst. Nanoeng., 2024, 10, 126 |
62. | A. C. Ericsson, M. J. Crim, C. L. Franklin, Mo Med, 2013, 110, 201 |
63. | K. R. Patil, U. B. Mahajan, B. S. Unger, S. N. Goyal, S. Belemkar, S. J. Surana, S. Ojha, C. R. Patil, Int. J. Mol. Sci., 2019, 20, 4376 |
64. | E. E. Patton, L. I. Zon, D. M. Langenau, Nat. Rev. Drug Discov., 2021, 20, 611 |
65. | U. B. Pandey, C. D. Nichols, Pharmacol. Rev., 2011, 63, 411 |
66. | R. S. Prather, M. Lorson, J. W. Ross, J. J. Whyte, E. Walters, Annu. Rev. Anim. Biosci., 2013, 1, 203 |
67. | K. A. Phillips, K. L. Bales, J. P. Capitanio, A. Conley, P. W. Czoty, B. A. t Hart, W. D. Hopkins, S. L. Hu, L. A. Miller, M. A. Nader, P. W. Nathanielsz, J. Rogers, C. A. Shively, M. L. Voytko, Am. J. Primatol., 2014, 76, 801 |
68. | J. L. Rowell, D. O. McCarthy, C. E. Alvarez, Trends Mol. Med., 2011, 17, 380 |
69. | G. L. Duff, M. G. Mc, A. C. Ritchie, Am J Pathol, 1957, 33, 845 |
70. | J. Nourinezhad, V. Rostamizadeh, R. Ranjbar, Ann. Anat., 2022, 242, 151911 |
71. | J. K. Lunney, A. Van Goor, K. E. Walker, T. Hailstock, J. Franklin, C. Dai, Sci. Transl. Med., 2021, 13, eabd5758 |
72. | N. B. Robinson, K. Krieger, F. M. Khan, W. Huffman, M. Chang, A. Naik, R. Yongle, I. Hameed, K. Krieger, L. N. Girardi, M. Gaudino, Int. J. Surg., 2019, 72, 9 |
73. | G. Qu, S. Wang, Z. Zhou, D. Jiang, A. Liao, J. Luo, Front. Immunol., 2022, 13, 891687 |
74. | P. Pound, S. Ebrahim, P. Sandercock, M. B. Bracken, I. Roberts, Bmj, 2004, 328, 514 |
75. | S. K. Doke, S. C. Dhawale, Saudi Pharm. J., 2015, 23, 223 |
76. | Y. Zeng, Z. Gu, Chinese Science Bulletin, 2023, 68, 4954 |
77. | D. Huh, G. A. Hamilton, D. E. Ingber, Trends Cell Biol., 2011, 21, 745 |
78. | L. A. Low, C. Mummery, B. R. Berridge, C. P. Austin, D. A. Tagle, Nat. Rev. Drug Discov., 2021, 20, 345 |
79. | J. Y. Shoji, R. P. Davis, C. L. Mummery, S. Krauss, Adv. Healthc. Mater., 2024, 13, e2301067 |
80. | H. Liu, Y. Wang, K. Cui, Y. Guo, X. Zhang, J. Qin, Adv. Mater., 2019, 31, e1902042 |
81. | G. Rossi, A. Manfrin, M. P. Lutolf, Nat. Rev. Genet., 2018, 19, 671 |
82. | M. Chen, H. Shan, Q. Tao, R. Hu, Q. Sun, M. Zheng, Z. Chen, Q. Lin, M. Yin, S. Zhao, X. Chen, Z. Chen, Small, 2024, 20, e2308525 |
83. | S. Wiedenmann, M. Breunig, J. Merkle, C. von Toerne, T. Georgiev, M. Moussus, L. Schulte, T. Seufferlein, M. Sterr, H. Lickert, S. E. Weissinger, P. Möller, S. M. Hauck, M. Hohwieler, A. Kleger, M. Meier, Nat. Biomed. Eng., 2021, 5, 897 |
84. | T. Tao, P. Deng, Y. Wang, X. Zhang, Y. Guo, W. Chen, J. Qin, Adv. Sci. (Weinh), 2022, 9, e2103495 |
85. | C. Quílez, E. Y. Jeon, A. Pappalardo, P. Pathak, H. E. Abaci, Adv. Healthc. Mater., 2024, 13, e2400405 |
86. | S. E. Park, A. Georgescu, D. Huh, Science, 2019, 364, 960 |
87. | V. S. Shirure, C. C. W. Hughes, S. C. George, Annu. Rev. Biomed. Eng., 2021, 23, 141 |
88. | A. G. Monteduro, S. Rizzato, G. Caragnano, A. Trapani, G. Giannelli, G. Maruccio, Biosens. Bioelectron., 2023, 231, 115271 |
89. | B. S. Elci, M. Nikolaev, S. Rezakhani, M. P. Lutolf, Adv. Healthc. Mater., 2024, 13, e2302912 |
90. | O. Mitrofanova, M. Nikolaev, Q. Xu, N. Broguiere, I. Cubela, J. G. Camp, M. Bscheider, M. P. Lutolf, Cell Stem Cell, 2024, 31, 1175 |
91. | N. Gjorevski, M. Nikolaev, T. E. Brown, O. Mitrofanova, N. Brandenberg, F. W. DelRio, F. M. Yavitt, P. Liberali, K. S. Anseth, M. P. Lutolf, Science, 2022, 375, eaaw9021 |
92. | H. Jian, X. Li, Q. Dong, S. Tian, S. Bai, Cell Prolif., 2023, 56, e13465 |
93. | V. Paloschi, J. Pauli, G. Winski, Z. Wu, Z. Li, L. Botti, S. Meucci, P. Conti, F. Rogowitz, N. Glukha, N. Hummel, A. Busch, E. Chernogubova, H. Jin, N. Sachs, H. H. Eckstein, A. Dueck, R. A. Boon, A. R. Bausch, L. Maegdefessel, Adv. Healthc. Mater., 2024, 13, e2302907 |
94. | Y. Jun, J. Lee, S. Choi, J. H. Yang, M. Sander, S. Chung, S. H. Lee, Sci. Adv., 2019, 5, eaax4520 |
95. | B. Waclawiková, A. Codutti, K. Alim, S. El Aidy, Gut Microbes, 2022, 14, 1997296 |
96. | A. Grassart, V. Malardé, S. Gobaa, A. Sartori-Rupp, J. Kerns, K. Karalis, B. Marteyn, P. Sansonetti, N. Sauvonnet, Cell Host Microbe, 2019, 26, 435 |
97. | K. H. Vining, D. J. Mooney, Nat. Rev. Mol. Cell Biol., 2017, 18, 728 |
98. | T. Mammoto, D. E. Ingber, Development, 2010, 137, 1407 |
99. | M. Niu, Y. Zhu, X. Ding, Y. Zu, Y. Zhao, Y. Wang, Adv. Healthc. Mater., 2023, 12, e2300850 |
100. | D. Xu, Y. Wang, L. Sun, Z. Luo, Y. Luo, Y. Wang, Y. Zhao, ACS Nano, 2023, 17, 15180 |
101. | Y. Zhu, L. Sun, Y. Wang, L. Cai, Z. Zhang, Y. Shang, Y. Zhao, Adv. Mater., 2022, 34, e2108972 |
102. | Y. Zhang, Y. Wang, H. Yin, J. Wang, N. Liu, S. Zhong, L. Li, Q. Zhang, T. Yue, Microsyst. Nanoeng., 2024, 10, 88 |
103. | K. Achberger, C. Probst, J. Haderspeck, S. Bolz, J. Rogal, J. Chuchuy, M. Nikolova, V. Cora, L. Antkowiak, W. Haq, N. Shen, K. Schenke-Layland, M. Ueffing, S. Liebau, P. Loskill, elife, 2019, 8, e46188 |
104. | T. A. V. Afanasyeva, J. C. Corral-Serrano, A. Garanto, R. Roepman, M. E. Cheetham, R. W. J. Collin, Cell Mol. Life Sci., 2021, 78, 6505 |
105. | E. Garreta, R. D. Kamm, S. M. Chuva de Sousa Lopes, M. A. Lancaster, R. Weiss, X. Trepat, I. Hyun, N. Montserrat, Nat. Mater., 2021, 20, 145 |
106. | R. Zandi Shafagh, S. Youhanna, J. Keulen, J. X. Shen, N. Taebnia, L. C. Preiss, K. Klein, F. A. Büttner, M. Bergqvist, W. van der Wijngaart, V. M. Lauschke, Adv. Sci. (Weinh), 2022, 9, e2203368 |
107. | V. V. T. Nguyen, S. Ye, V. Gkouzioti, M. E. van Wolferen, F. Y. Yengej, D. Melkert, S. Siti, B. de Jong, P. J. Besseling, B. Spee, L. J. W. van der Laan, R. Horland, M. C. Verhaar, B. W. M. van Balkom, J. Extracell. Vesicles, 2022, 11, e12280 |
108. | S. Bauer, C. Wennberg Huldt, K. P. Kanebratt, I. Durieux, D. Gunne, S. Andersson, L. Ewart, W. G. Haynes, I. Maschmeyer, A. Winter, C. Ämmälä, U. Marx, T. B. Andersson, Sci. Rep., 2017, 7, 14620 |
109. | D. Bovard, A. Sandoz, K. Luettich, S. Frentzel, A. Iskandar, D. Marescotti, K. Trivedi, E. Guedj, Q. Dutertre, M. C. Peitsch, J. Hoeng, Lab Chip, 2018, 18, 3814 |
110. | J. W. Jeon, S. H. Lee, D. Kim, J. H. Sung, Biotechnol. Prog., 2021, 37, e3121 |
111. | D. N. Tavakol, T. R. Nash, Y. Kim, P. L. Graney, M. Liberman, S. Fleischer, R. I. Lock, A. O'Donnell, L. Andrews, D. Ning, K. Yeager, A. Harken, N. Deoli, S. A. Amundson, G. Garty, K. W. Leong, D. J. Brenner, G. Vunjak-Novakovic, Adv. Sci. (Weinh), 2024, 11, e2401415 |
112. | R. Janssen, J. W. M. de Kleer, B. Heming, S. Bastiaan-Net, J. Garssen, L. E. M. Willemsen, R. Masereeuw, Trends Biotechnol., 2024, 42, 119 |
113. | J. H. Sung, Y. I. Wang, N. Narasimhan Sriram, M. Jackson, C. Long, J. J. Hickman, M. L. Shuler, Anal. Chem., 2019, 91, 330 |
114. | M. B. Esch, G. J. Mahler, T. Stokol, M. L. Shuler, Lab Chip, 2014, 14, 3081 |
115. | Y. Suhail, M. P. Cain, K. Vanaja, P. A. Kurywchak, A. Levchenko, R. Kalluri, Kshitiz, Cell Syst., 2019, 9, 109 |
116. | N. Del Piccolo, V. S. Shirure, Y. Bi, S. P. Goedegebuure, S. Gholami, C. C. W. Hughes, R. C. Fields, S. C. George, Adv. Drug Deliv. Rev., 2021, 175, 113798 |
117. | D. Caballero, S. Kaushik, V. M. Correlo, J. M. Oliveira, R. L. Reis, S. C. Kundu, Biomaterials, 2017, 149, 98 |
118. | Z. Zou, Z. Lin, C. Wu, J. Tan, J. Zhang, Y. Peng, K. Zhang, J. Li, M. Wu, Y. Zhang, Adv. Sci. (Weinh), 2023, 10, e2302640 |
119. | C. Ma, Y. Peng, H. Li, W. Chen, Trends Pharmacol. Sci., 2021, 42, 119 |
120. | J. Huang, Z. Xu, J. Jiao, Z. Li, S. Li, Y. Liu, Z. Li, G. Qu, J. Wu, Y. Zhao, K. Chen, J. Li, Y. Pan, X. Wu, J. Ren, Bioact. Mater., 2023, 30, 1 |
121. | Y. Zhu, D. Jiang, Y. Qiu, X. Liu, Y. Bian, S. Tian, X. Wang, K. J. Hsia, H. Wan, L. Zhuang, P. Wang, Bioact. Mater., 2024, 39, 59 |
122. | M. Zhang, P. Wang, R. Luo, Y. Wang, Z. Li, Y. Guo, Y. Yao, M. Li, T. Tao, W. Chen, J. Han, H. Liu, K. Cui, X. Zhang, Y. Zheng, J. Qin, Adv. Sci. (Weinh), 2021, 8, 2002928 |
123. | V. Negi, D. Gavlock, M. T. Miedel, J. K. Lee, T. Shun, A. Gough, L. Vernetti, A. M. Stern, D. L. Taylor, V. K. Yechoor, Lab Chip, 2023, 23, 4514 |
124. | D. Butler, D. R. Reyes, Lab Chip, 2024, 24, 1494 |
125. | S. Park, T. C. Laskow, J. Chen, P. Guha, B. Dawn, D. H. Kim, Aging Cell, 2024, 23, e14070 |
126. | W. Balestri, R. Sharma, V. A. da Silva, B. C. Bobotis, A. J. Curle, V. Kothakota, F. Kalantarnia, M. V. Hangad, M. Hoorfar, J. L. Jones, M. Tremblay, J. J. El-Jawhari, S. M. Willerth, Y. Reinwald, J Neuroinflammation, 2024, 21, 32 |
127. | J. Ko, J. Song, Y. Lee, N. Choi, H. N. Kim, Lab Chip, 2024, 24, 1542 |
128. | B. J. Kwee, X. Li, X. X. Nguyen, C. Campagna, J. Lam, K. E. Sung, Exp. Biol. Med. (Maywood), 2023, 248, 2001 |
129. | K. Ge, Y. Ren, Z. Hong, Z. Mao, B. Yao, K. Ye and C. Jia, Adv. Healthc. Mater., 2024, 13, e2401990 |
130. | L. H. Goetz, N. J. Schork, Fertil. Steril., 2018, 109, 952 |
131. | P. C. Beltrão-Braga, G. C. Pignatari, F. B. Russo, I. R. Fernandes, A. R. Muotri, Cytometry A, 2013, 83, 11 |
132. | Y. A. Jodat, M. G. Kang, K. Kiaee, G. J. Kim, A. F. H. Martinez, A. Rosenkranz, H. Bae, S. R. Shin, Curr Pharm Des, 2018, 24, 5471 |
133. | E. W. Esch, A. Bahinski, D. Huh, Nat. Rev. Drug Discov., 2015, 14, 248 |
134. | U.S. Food and Drug Administration (FDA), FDA Voice: Organs-on-Chips Technology, https://wayback.archive-it.org/8521/20180903192020/https://blogs.fda.gov/fdavoice/index.php/tag/organs-on-chips-technology/, September 2018. |
135. | U.S. Food and Drug Administration (FDA), Advancing New Alternative Methodologies at FDA, https://www.fda.gov/media/144891/download, January 2021. |
136. | Valuates Reports, Global Organs-on-Chips (OOC) Market Report, https://reports.valuates.com/market-reports/QYRE-Auto-9N6280/global-organs-on-chips-ooc, 2024. |
137. | S. Deng, C. Li, J. Cao, Z. Cui, J. Du, Z. Fu, H. Yang, P. Chen, Theranostics, 2023, 13, 4526 |
138. | M. Busek, S. Nøvik, A. Aizenshtadt, M. Amirola-Martinez, T. Combriat, S. Grünzner, S. Krauss, Biosensors (Basel), 2021, 11, 162 |
139. | M. X. Doss, A. Sachinidis, Cells, 2019, 8, 403 |
140. | T. Ching, Y. C. Toh, M. Hashimoto, Y. S. Zhang, Trends Pharmacol. Sci., 2021, 42, 715 |
This is an open access article under the terms of the Creative Commons Attribution License, which permits use, distribution and reproduction in any medium, provided the original work is properly cited.
The schematic diagram of organ-on-chips. Various OOCs for the human body are introduced in the outer circle, three applications of OOC are introduced on the left side of the inner circle, and three ways of OOC fabrication are introduced on the right side. Source: Created with BioRender.com.
This picture introduces four tools for preclinical drug screening and disease modeling: 2D cell culture, 3D cell culture and organ-on-chip models in vitro, and small and large animal models such as mice and pigs in vivo. Source: Created with BioRender.com.
a (i) Schematic diagram of the TOP system's capabilities; (ii) Schematic of the distribution of Matrigel in the TOP; (iii) Schematic illustration of the cytokine concentration gradient in the TOP.[82] Copyright 2024, Wiley-VCH GmbH. b Schematic of hiPSCs derived multi-organoid-on-chip system in vitro. (i) Illustration of how human liver and pancreatic islet tissues regulate glucose levels in vivo; (ii) The differentiation, formation, and co-culture process of liver and pancreatic islet organoids derived from hiPSCs in the microwell device; (iii) Microfluidic perfusion system device and chip physical diagram.[84] Copyright 2021, The Authors. Advanced Science published by Wiley-VCH GmbH. c (i) Schematic representation of PSO culture within a microfluidic system; (ii) Analysis of the chemical gradient for molecules of A) high (40 kDa) and B) low (519 Da) molecular weight; (iv) IF images of Krt14 (in red) in PSO cultures under different conditions on day 32 within the device.[85] Copyright 2024, Wiley-VCH GmbH.
a The formation of the complex biliary tree within prepatterned hydrogel chips. (i) Laser-ablated channel diameter of the branch network is from 150 to 50 μm; (ii) Time-lapse bright field images depicting the epithelium formation following repopulating by organoid-derived cholangiocytes from d0 (left) to d7 (right); (iii) Live-imaging of Rhodamine 123 in the structure at 10 days from delivery; (iv) 3D reconstruction of the entire construct, along with three distinct views on day 8, showing Nuclei and F-actin staining.[89] Copyright 2024, Wiley-VCH GmbH. b (i) Numerical simulation comparing the O2 concentration profiles within PSOs grown in 3D ECM as a sphere versus as a spindle shape in the spindle device; (ii) On day 32, IF images of Caspase 3 and DAPI in PSO.[85] Copyright 2024, Wiley-VCH GmbH. c (i) Schematic representation of the workflow for generating human mini-colons; (ii) Laser ablation creates an open microchannel with specific dimensions; (iii) Bright-field time-course imaging of the formation of epithelium in mini-colons.[90] Copyright 2024, The Author(s). Published by Elsevier Inc. d (i) The generation process of microfabricated tissues; (ii) A collection of intestinal organoids derived from engineered intestinal tissues with a rod-like shape and specified magnification; (iii) Frequency map of Lgr 5, showing average Lgr 5 expression over around 80 tissues; (iv) Lysozyme staining of Paneth cells in the array of intestinal organoids; v) average Paneth cell distribution; (vi) 3D reconstruction of the immunofluorescence images.[91] Copyright 2022, The American Association for the Advancement of Science. e (i) Illustration of the in vitro fabrication of liver organoids featuring biomimetic lobular architecture through a multi-cellular 3D bioprinting approach; (ii) Programming of 3D droplet-based bioprinting for the in vitro liver model fabrication; (iii) Multicellular composition of the assembled liver organoid; (iv) Printing process photos; 3D liver organoid and its hydrogel scaffold optical image; confocal microscopy image showing the hexagonal central region within the lobule-like structure following 1 day of culture; (v) Confocal images of the assembled liver organoids following 7 days of culture; (vi) Cell proliferation assay results.[92] Copyright 2023, The Authors. Cell Proliferation published by Beijing Institute for Stem Cell and Regenerative Medicine and John Wiley & Sons Ltd.
a (i) Cross-sectional design of AoC model; (ii) EC, SMC co-culture membrane schematic diagram; (iii) Schematic diagram of AoC and microfluidic pump; (iv) 3D view of the bottom channel; (v) 2D diagram of wall shear stress distribution along film; (vi) Immunofluorescence staining of EC and SMC on the membrane.[93] Copyright 2023, The Authors. Advanced Healthcare Materials published by Wiley-VCH GmbH. b Spheroid-based microfluidic perfusion culture of pancreatic islets used to simulate the in vivo environment. (i) Clusters of endocrine cells are scattered throughout the exocrine acini in the native pancreas, forming islets of Langerhans; (ii) Engineering of islet spheroids on a microchip with a perfusion system is implemented to simulate in vivo environment; (iii-v) Schematics of the experimental setup; (vi) Pancreatic islet cells cultured in microfluidic chips under three disparate environments.[94] Copyright 2019, The American Association for the Advancement of Science. c Progression and characterization of human ecto-and endo-cervix chips. (i) Schematic diagram and cross-sectional design of two-channel microfluidic organ chip; (ii) A fluorescent microscopy side view shows the mucous layer in live Cervix Chip cultures, stained with wheat germ agglutinin (WGA) fluorescence (green), after seven days of differentiation. The images compare the effects of continuous flow (top) and periodic flow (bottom) regimens (bar, 1 mm); (iii) Phase-contrast microscopy top view of a Cervix Chip, with cervical epithelium on the apical side and fibroblasts on the basal side of the porous membrane, captured on day 1 (left) and after 12 days of culture under continuous (middle) or periodic (right) flow conditions (bar, 200 μm); (iv) Cervical epithelial cells’ gene expression from three different donors were RNAseq analyzed and differentiated into static Transwell or Cervix chips in either a periodic or continuous flow.[20] Copyright 2024, The Author(s).
a (i-ii) Schematic illustration of a simulated alveolar device with IOS-PU film; (iii-vi) Characterization of alveolar-mimicking devices; (iii-iv) The relationship between tensile degree and perfusion volume; (v) The relationship between tensile degree and film color; (vi) Changes of reflection spectra of IOS-PU films under different stretching degree.[99] Copyright 2023, Wiley-VCH GmbH. b (i) Schematic representation of the living anisotropic structural color hydrogels used for screening cardiotoxicity caused by environmental toxins; (ii) Heart-on-a-chip platform; (iii) Optical microscopic images showing structural color variations throughout a beating cycle of hiPSC-derived cardiomyocytes (hiPSC-CMs); (iv-v) The CLSM images of hiPSC-CMs treated with 75 μg/mL PQ (iv) and 25 μM CdCl2 (v) were sustained for 24 hours when anisotropic structural color hydrogels in vivo were evaluated for environmental toxins.[100] Copyright 2023, American Chemical Society. c (i-ii) Principle and schematic diagram of bionic human lung chip with microphysiological respiration visualization; (iii-vi) Engineered pulmonary alveolus deform under low and high cycle stretching; Low (iii-iv) and high (v-vi) flow PDMS film deformation diagram and numerical simulation of film deformation at peak cycle; (vii) Schematic of idiopathic pulmonary fibrosis (IPF) model by exposure to TGF-β; (viii-ix) Optical microscope images of structural color changes of low and high airflow during breathing under IPF model; (x) EdU staining (red) of co-cultured HFL1 and HPAEpiC cells, with or without cyclic stretching after TGF-ββ treatment.[101] Copyright 2022, Wiley-VCH GmbH.
a (i) Pneumatic hybrid microfluidic device; (ii) Schematic diagram of molecular networks interacting in prediabetic hyperglycemia; (iii) Pancreatic islets and liver spheroids are generated and recovered using ultralow attachment (ULA) plates; (iv-v) Immunofluorescent staining of primary human islets for insulin (β-cells), glucagon (α-cells), and somatostatin (δ-cells); (vi) Quantification of insulin secretion following exposure of islets to hypoglycemic or hyperglycemic conditions.[106] Copyright 2022, The Authors. Advanced Science published by Wiley-VCH GmbH. b (i-iii) Characterization of renal tubule. (i) photographs; (ii) cross-sectional view; (iii) schematic illustration; (iv) Immunofluorescence staining of 2D-culture renal tubuloids in static, dynamic, and dynamic co-culture with DAPI-stained nuclei (blue), the tight junction protein ZO-1 (green), and the Na/K-ATPase (red) showing polarized epithelial cell layers in all conditions; (v) Static, dynamic, and dynamic co-culture conditions of liver organoids; (vi) Experimental timeline. KDM: kidney differentiation medium, LDM: liver differentiation medium.[107] Copyright 2022, The Authors. Journal of Extracellular Vesicles published by Wiley Periodicals, LLC on behalf of the International Society for Extracellular Vesicles. c Multi-organ chip of bone marrow-heart-liver-vascular.[111] Copyright 2024, The Author(s). Advanced Science published by Wiley-VCH GmbH. d (i) A compartmentalized and functional gut-immune-skin OOC device; (ii) Legend for (i).[112] Copyright 2023, Author(s). Published by Elsevier Ltd.
a (i) Schematic of the absorption process of oral anti-lung cancer drugs and construction of a high-throughput intestine-liver-heart-lung cancer microphysiological system, which can evaluate four different drugs at the same time; (ii) Application of MSCP platform; (iii) The schematic representation of the organ formation mode.[121] Copyright 2024, The Authors. Publishing services by Elsevier B.V. on behalf of KeAi Communications Co. Ltd. b (i) 3D human alveolar-capillary barrier model in vivo; (ii) Configuration of a bionic human alveolus chip that is infected by SARS-CoV-2.[122] Copyright 2020, The Authors. Advanced Science published by Wiley-VCH GmbH. c (i) Schematic diagram of microfluidic chip architecture; (ii-vi) Application of PT-EVs in homologous tumor therapy.[129] Copyright 2024, Wiley-VCH GmbH.